Generative AI, a subset of artificial intelligence that can create new data like text, images, code, and more, is rapidly transforming the landscape of knowledge work. This cutting-edge technology leverages machine learning models trained on vast datasets to generate human-like content, revolutionizing how knowledge is created, shared, and applied across industries.
Knowledge work, which encompasses professions that primarily involve creating, synthesizing, and applying knowledge, has long been considered a domain where human intelligence reigns supreme. However, the advent of generative AI is challenging this notion, offering powerful tools that can augment and enhance knowledge workers' capabilities in unprecedented ways.
From drafting and editing documents to generating insights from data, designing products, and creating personalized content experiences, generative AI is poised to become an indispensable aid for knowledge workers across diverse fields such as research, marketing, product development, and customer service. As this technology continues to advance, it promises to reshape the very nature of knowledge work, ushering in a new era of human-machine collaboration and augmented intelligence.
Applications and Use Cases
A. Natural Language Processing and Generation
One of the core capabilities of generative AI for knowledge work is natural language processing (NLP) and generation. Large language models like GPT-3 can understand and generate human-like text with remarkable fluency and coherence. For knowledge workers, this translates into several powerful applications:
- Writing Assistance: Drafting documents like reports, articles, emails, Editing and refining written content, Summarizing long-form text into concise overviews.
- Information Extraction and Synthesis: Analyzing large volumes of text data, identifying key insights, trends and patterns, generating new knowledge by combining information from multiple sources.

By leveraging NLP, generative AI can significantly augment writing, research, and analytical capabilities of knowledge workers across industries like consulting, law, finance, and academia.
B. Multimodal Content Generation
Beyond text, generative AI is also capable of creating multimodal content spanning images, videos, code, and data visualizations based on text prompts or inputs.
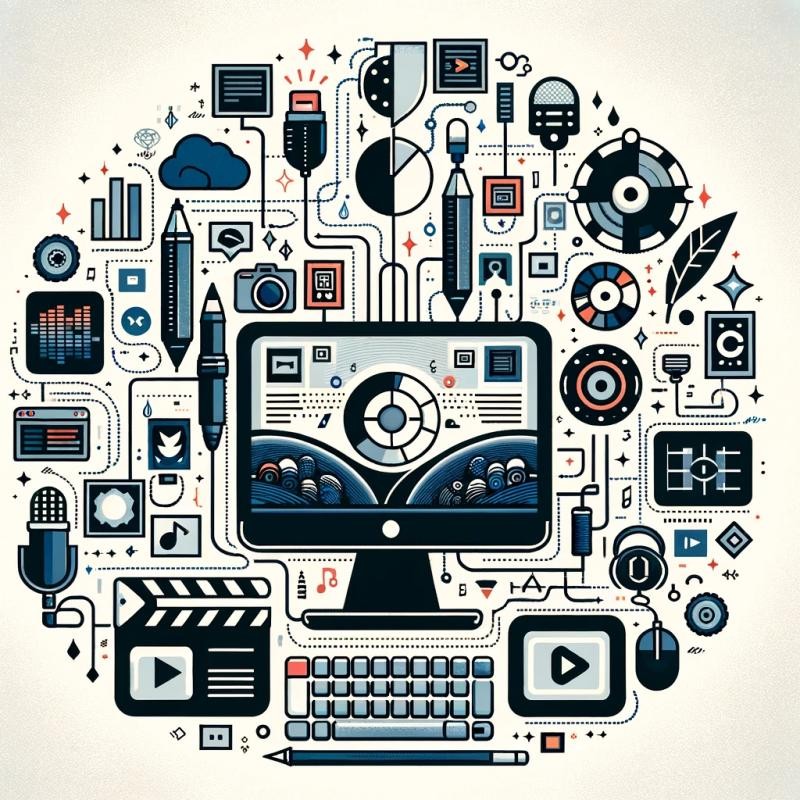
- Text-to-Image Generation: Creating custom images from text descriptions, Generating visual concepts, product designs, graphics.
- Code Generation: Automating software development tasks, Generating code snippets, functions, scripts.
- Data Visualization: Producing charts, graphs, infographics from data, Explaining complex data through visuals.
- Text-to-Audio or Video Generation: Creating custom training videos or podcast or internal multi-media videos
For knowledge workers, these multimodal capabilities can streamline creative ideation, prototyping, data analysis, and communication of complex ideas.
C. Personalized and Interactive Experiences
Generative AI also enables highly personalized and interactive user experiences by understanding and responding to natural language queries in context.
- Conversational AI Assistants: Answering questions, providing recommendations, Assisting with research, analysis, task planning
- Tailored Content Recommendations: Surfacing relevant information and resources, Customized learning paths, training materials.
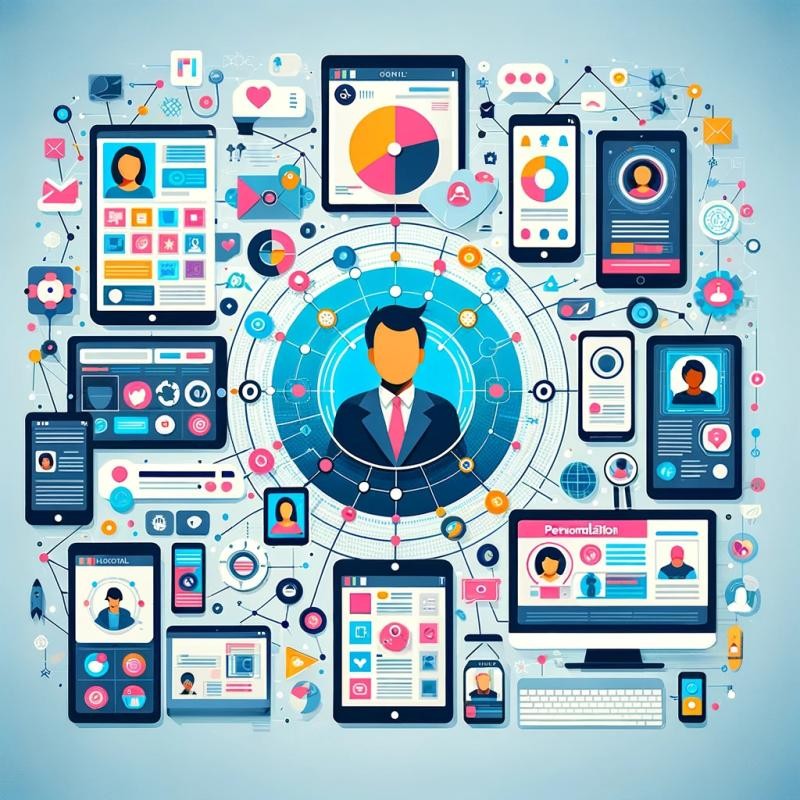
By engaging in back-and-forth dialogue, these AI assistants can serve as knowledgeable copilots, enhancing productivity and learning for knowledge workers.
Challenges and Considerations
Ethical Concerns
Generative AI raises ethical issues around privacy, security, deepfakes, misinformation, and intellectual property violations. Clear governance frameworks are needed for responsible development and use.
Human-AI Collaboration and Workforce Impact
Integrating generative AI will require carefully balancing human-AI collaboration to enhance rather than replace human expertise. Reskilling workforces to work alongside AI is crucial.
Data Quality and Bias
Biases and inaccuracies in training data can lead to generation of misinformation and lack of inclusivity in AI outputs, requiring careful data curation.
Regulatory and Legal Implications
As use grows, there will be a need for regulations addressing liability, accountability, data privacy, and ethical AI governance in knowledge work contexts.
Proactively addressing these ethical, workforce, data quality, and regulatory challenges is key to mitigating risks and realizing generative AI's full potential for augmenting knowledge workers.
Best Practices and Future Outlook
As generative AI capabilities rapidly advance, it is crucial to prioritize responsible and ethical development practices from the outset. This includes:
- Ensuring diverse and representative training data to mitigate biases.
- Implementing robust testing and monitoring for safety and reliability.
- Promoting transparency by documenting model capabilities and limitations.
- Establishing clear guidelines for appropriate use cases and human oversight.
Upskilling and Reskilling for the AI-Augmented Workforce
To fully leverage generative AI's potential, organizations must invest in upskilling and reskilling their knowledge workers. Key focus areas include:
- Data literacy and ability to work with AI models.
- Creative and strategic thinking to guide AI-augmented work.
- Developing "human skills" like emotional intelligence and critical thinking.
Continuous learning programs and skills-based hiring will be essential for cultivating an AI-ready workforce.
Emerging Trends and Future Applications
Generative AI is still in its nascent stages, with new breakthroughs and applications emerging rapidly. Some key trends to watch include:
- Multimodal models combining text, images, audio, and sensor data
- Conversational AI assistants with deeper context and reasoning abilities
- AI-powered automation of increasingly complex knowledge work tasks
- Integration of generative AI into enterprise software and workflows

The rise of generative AI represents a paradigm shift in how knowledge work is performed. While challenges exist, responsible adoption guided by best practices can unlock immense potential for augmenting human intelligence.
Ultimately, generative AI is poised to be a pivotal force driving innovation and productivity across knowledge-intensive industries.