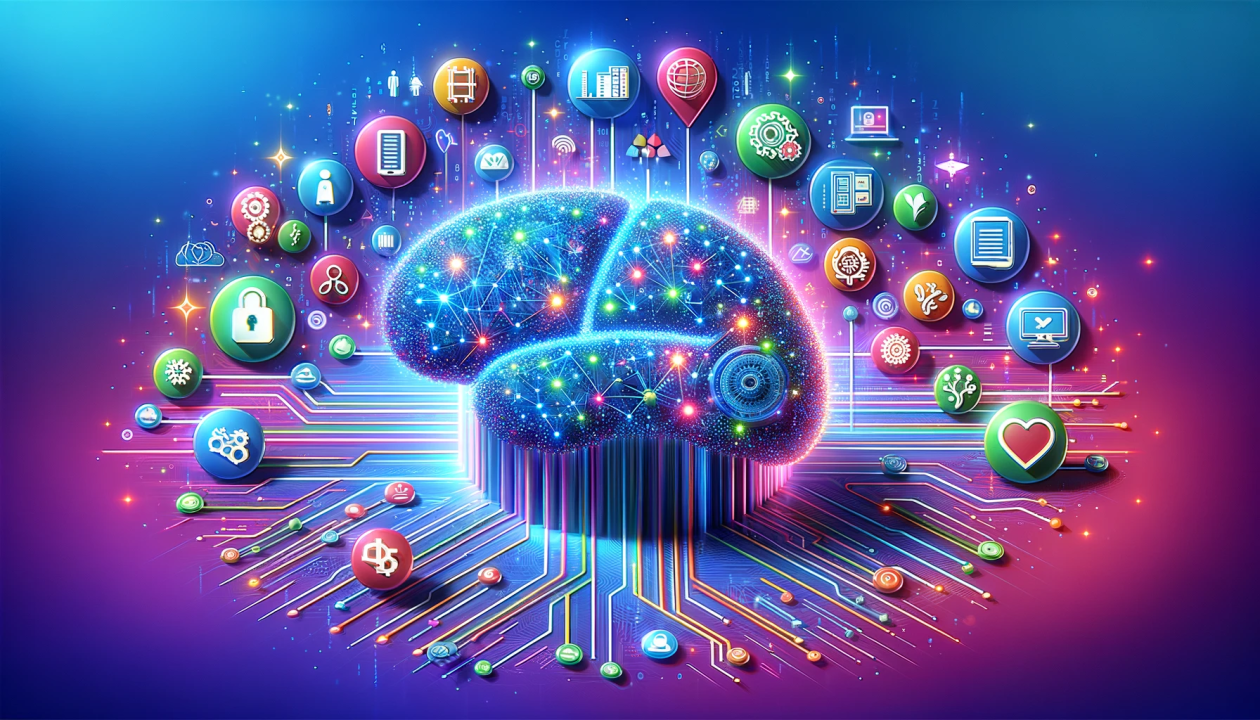
In today's rapidly evolving world of AI, large language models (LLMs) have emerged as a powerful tool for processing and analyzing vast amounts of natural language data. As the demand for AI-driven solutions increases, understanding the importance and applications of LLMs is crucial for businesses and individuals alike. This article will provide an overview of LLMs, their use cases, and how they can be integrated into various domains to enhance communication, automate tasks, and drive innovation.
Large Language Models (LLMs) represent a groundbreaking stride in artificial intelligence, particularly in the realm of natural language processing. These sophisticated models, leveraging vast amounts of data, have the remarkable ability to understand, interpret, and generate human-like text. LLMs have transformed how machines interact with human language, making them integral in various applications, from automated customer service to content creation. Their increasing accuracy and efficiency signify a major shift in technology, heralding new possibilities in AI-human interaction. This introduction to LLMs will shed light on their fundamental aspects and growing importance in today's digital landscape.
The evolution of Large Language Models traces back to the inception of computational linguistics, where early models relied heavily on rule-based systems. Over time, these evolved into more sophisticated, data-driven approaches, fueled by advancements in machine learning and artificial intelligence. The leap from simple, syntax-based algorithms to complex models capable of understanding context and nuances in language marks a significant milestone in this journey. These developments paved the way for the current generation of LLMs, which are not only more efficient but also exhibit an unprecedented level of fluency and understanding in human language processing. This historical context sets the stage for appreciating the complexity and capabilities of modern LLMs. Let's review some key milestones:
1950 - 1990 Early Rule-Based Systems: The initial phase where language models relied on fixed rules for language processing.
2002s - Introduction of Machine Learning: A significant shift from rule-based to data-driven approaches using statistical methods.
2012 - Neural Network Revolution: The adoption of neural networks, particularly deep learning, greatly enhanced the language models' capabilities.
2018 - Development of Transformer Models: Introduction of transformer architecture, leading to models like GPT and BERT, which significantly improved context understanding and fluency.
2022 - Recent Advances and Scalability: The recent period has seen models becoming more scalable and efficient, handling more complex tasks and larger datasets.
Delving into the workings of Large Language Models is an exhilarating journey into AI's heart. These models learn from vast datasets including the entire content on the world wide web, absorbing the nuances and complexities of human language. They use intricate algorithms to predict and generate text "token", mimicking human-like understanding and expression. This incredible ability to process and produce language in a contextually relevant manner is transforming how we interact with technology, opening doors to endless possibilities in communication and information processing. The magic of LLMs lies in their deep learning architectures, making them not just tools, but partners in our digital world.
This revolution started with the raise of the transformer architecture. A transformer is a type of neural network architecture that has revolutionized the field of natural language processing. Unlike previous models that processed words in sequence, transformers can look at entire sequences of words at once, enabling them to understand context more effectively. This capability allows transformers to excel in tasks like translation, summarization, and question-answering. For example, in a translation task, a transformer can consider the entire sentence, understanding both the meaning and the grammatical structure, to produce a more accurate and coherent translation in another language.
The journey of Large Language Models into the public spotlight reached a pivotal moment with the release of ChatGPT in November 2022. This event marked a significant leap forward, showcasing the advanced capabilities of LLMs in engaging in human-like conversations. ChatGPT's ability to understand context, provide detailed responses, and even exhibit elements of creativity captured global attention. Its widespread adoption and media coverage highlighted the practical and innovative potential of LLMs, fueling public interest and discussion about the future of AI in everyday life. This milestone not only elevated the status of LLMs but also set a new benchmark for interactive AI technology.
In less than a few years, LLM have found remarkable applications across diverse industries, showcasing their versatility and impact.
The advent of ChatGPT, a proprietary model, significantly influenced the landscape of language models, paving the way for a surge in open-source alternatives. These open-source models offer a key advantage in terms of transparency, allowing users and developers to understand and modify their inner workings. This shift is critical for fostering innovation, trust, and broader accessibility in the field of AI.
By enabling more hands-on exploration and customization, open-source LLMs are democratizing the field, empowering smaller entities and individuals to contribute to and benefit from these advanced technologies. The coexistence of proprietary and open-source models presents a dynamic ecosystem, where choice and diversity drive progress and ethical considerations.
As we look towards the future, Large Language Models stand at the forefront of a major technological revolution. Their evolving capabilities hint at a future where AI and human interaction become more seamless and intuitive.
LLMs will likely penetrate deeper into various sectors, enhancing efficiency and enabling new forms of creativity and problem-solving. However, this progress brings with it ethical and practical challenges that need addressing, particularly in areas of data privacy, transparency, security, and bias.
The ongoing development and integration of LLMs will undoubtedly shape the technological landscape, influencing how we work, learn, and communicate. Their impact extends beyond mere tools, potentially redefining our relationship with technology.